Dynamic and Low-Rank Fine-Tuning of Large Language Models for Robust Few-Shot Learning
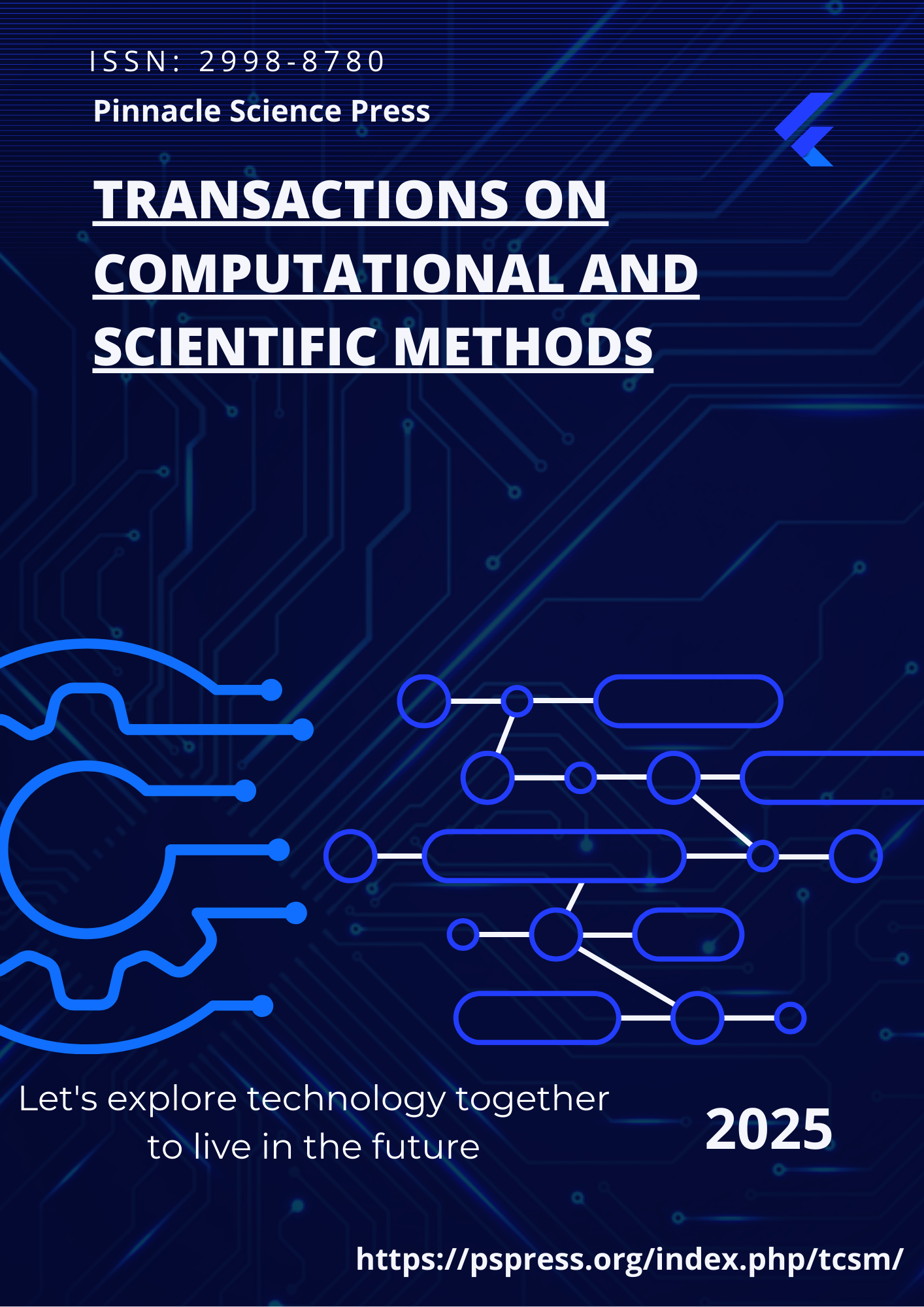
Abstract
This paper focuses on the fine-tuning of large models in data-scarce scenarios and proposes a parameter-efficient fine-tuning strategy that combines low-rank decomposition with dynamic weight adjustment to enhance model adaptability and stability under few-shot conditions. To address the high computational cost and overfitting risks associated with traditional full-parameter fine-tuning, the proposed method leverages the LoRA structure to reduce the number of trainable parameters and introduces a dynamic weighting mechanism based on sample uncertainty, guiding the model to focus more on high-value samples. This improves the model's generalization ability while preserving its representational capacity. Experiments conducted on the FewRel few-shot relation classification dataset demonstrate that the proposed method achieves higher accuracy and F1 scores than full fine-tuning while updating only 1.5% of the parameters, significantly improving training efficiency and robustness. Furthermore, a sensitivity analysis of frozen layers confirms the strategy’s ability to strike a balance between parameter control and transfer performance. The dynamic weight adjustment mechanism also consistently shows notable performance gains across different K-shot settings, indicating its strong value for training optimization in data-scarce environments.