Transformer-Based Structural Anomaly Detection for Video File Integrity Assessment
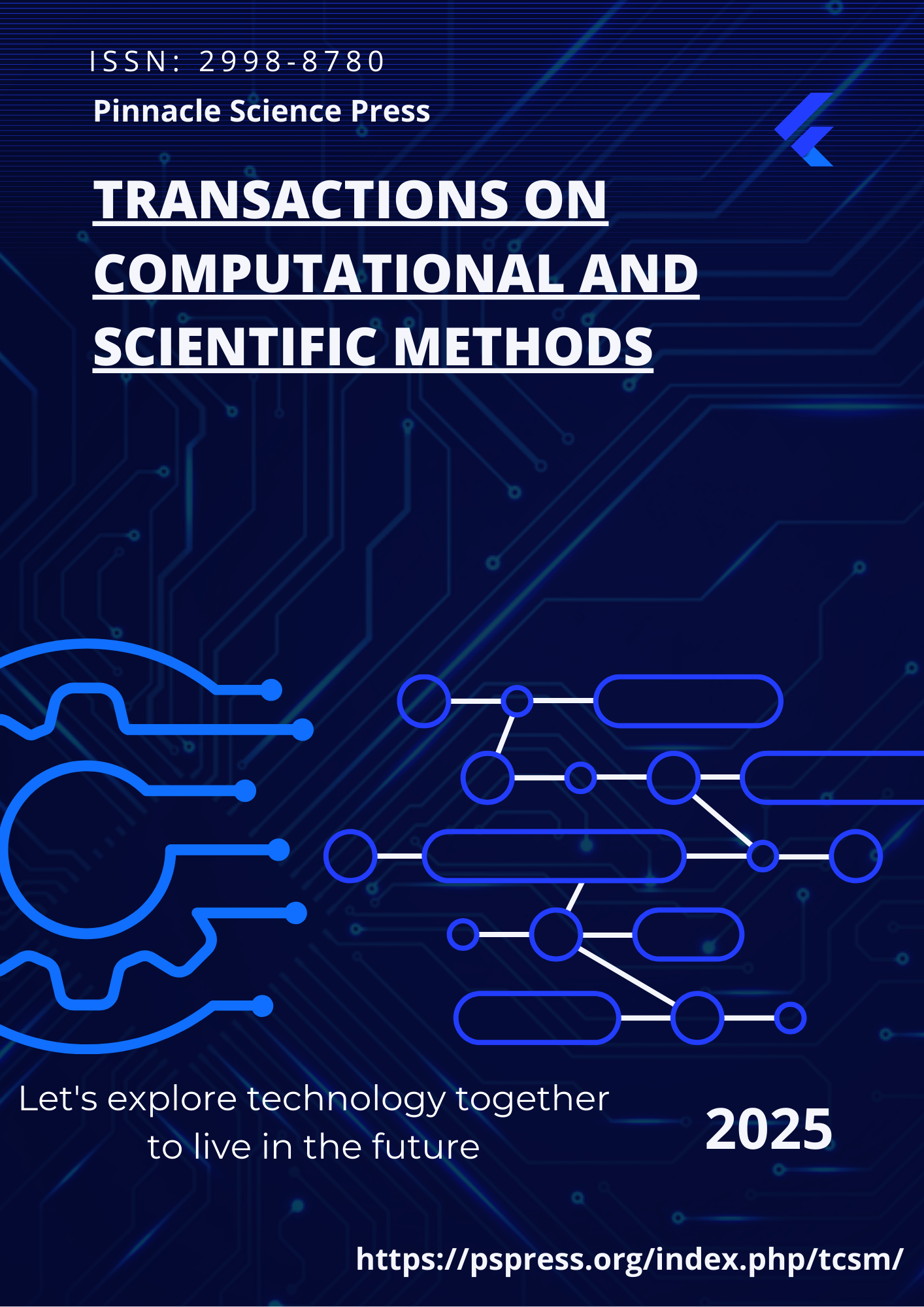
Abstract
With the widespread use of video data in surveillance, security, and communication, the integrity of video files has become a key factor affecting data usability and trustworthiness. Compared to semantic analysis of video content, structural anomalies—such as frame loss, container errors, timestamp disorder, and bitstream corruption—have a more direct impact on video parsing and usage. However, existing methods often rely on rule-based checks or content-driven learning strategies, making them less robust for complex structural anomalies. To address this, we propose a Transformer-based method for video structural integrity anomaly detection. It employs structural embeddings and hierarchical attention to build multi-scale representations of video files, effectively identifying hidden anomalies in containers, frame sequences, and encoding parameters. The method takes structural metadata sequences as input and performs global modeling and local refinement using a multi-stage Swin Transformer architecture, producing corresponding integrity scores. Experiments on video datasets show that our method outperforms mainstream models in terms of detection accuracy, recall, and anomaly recognition stability, while maintaining strong generalization and execution efficiency across encoding formats and under low-resource conditions. This study not only introduces a novel modeling approach for video integrity analysis but also provides critical technical support for quality control and security protection in multimedia systems.