Distributed Network Traffic Scheduling via Trust-Constrained Policy Learning Mechanisms
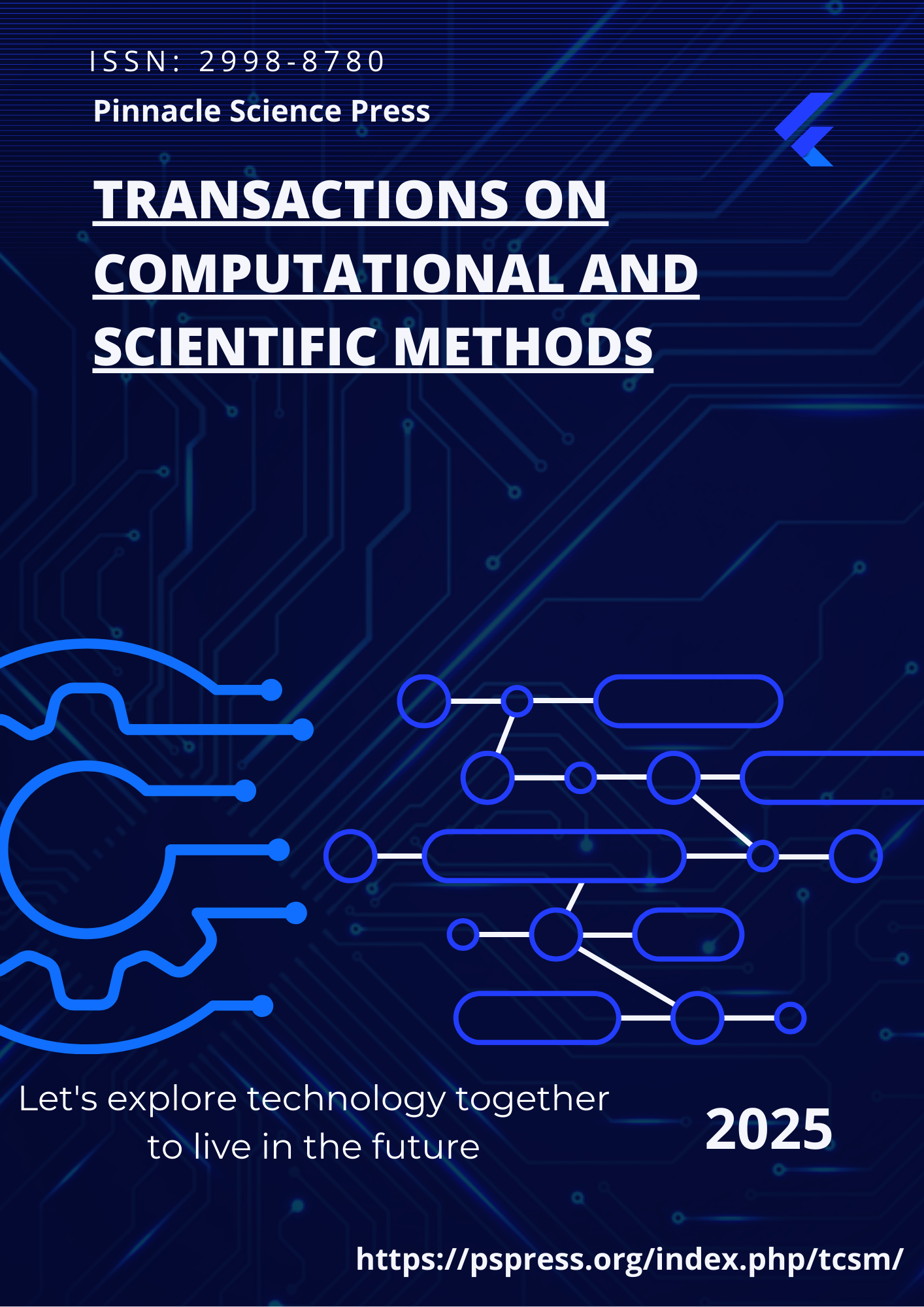
Abstract
This paper proposes a distributed network traffic scheduling optimization method based on Trust Region Policy Optimization (TRPO) to improve the utilization rate of network resources and reduce network congestion. Experiments on Abilene network traffic data sets demonstrate that, compared with traditional reinforcement learning methods such as Deep Q-Networks (DQN) and Proximal Policy Optimization (PPO) , TRPO achieves superior scheduling performance under varying traffic load conditions, effectively reduces the maximum link congestion rate, optimizes average delay, and exhibits strong robustness in burst traffic scenarios. The findings underscore the significance of integrating advanced artificial intelligence techniques, particularly policy-based reinforcement learning, into the realm of network traffic management. This contribution holds critical importance for industries such as telecommunications, cloud computing, and data center operations, where real-time traffic optimization is essential for maintaining service quality and operational efficiency. By enabling more intelligent, adaptive, and stable distributed scheduling, this approach not only advances the state of AI-driven networking but also lays the foundation for scalable and generalizable solutions. Future research combining multi-agent reinforcement learning, graph neural networks, and other AI technologies can further enhance the scalability and applicability of traffic scheduling systems, fostering transformative improvements in intelligent network infrastructure across key sectors.