Default Risk Prediction for Small and Micro Enterprises Using a Wide and Deep Learning Framework
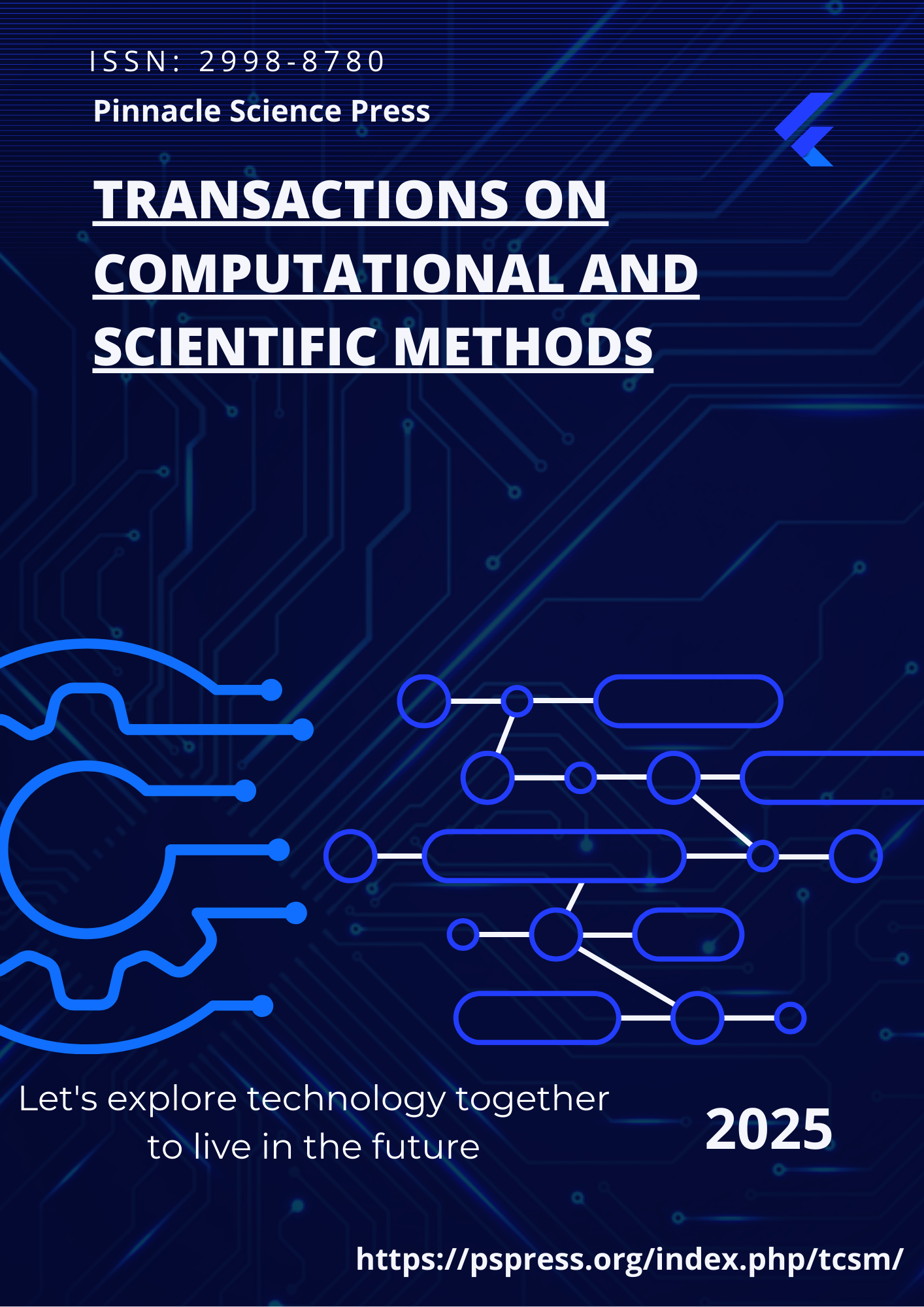
Abstract
Delinquency risk prediction is a core component of credit loan operations, directly impacting the profitability of lending institutions and the control of bad debt. With the rapid development of mobile internet, credit services have become widely accessible to the general public. Traditional risk control methods based on manual rule-making are gradually being replaced by data-driven intelligent modeling techniques. Existing approaches mainly include traditional machine learning models and deep learning models. The former offers strong interpretability but limited predictive performance, while the latter excels in prediction accuracy but suffers from weak interpretability and a high risk of overfitting. To balance the strengths of both, this paper draws inspiration from the Wide & Deep model architecture commonly used in recommendation systems and proposes a hybrid modeling framework that combines logistic regression with deep neural networks. This framework is designed to extract key features from both structured and unstructured data and to predict the probability of delinquency through a unified linear layer. Specifically, the wide component utilizes logistic regression to process structured variables such as basic enterprise information and cross features, while the deep component employs a three-layer fully connected neural network to handle unstructured data such as transaction flows. Through end-to-end training, the model simultaneously optimizes both components, thereby enhancing overall performance. Experiments conducted on a real-world credit dataset for small and micro enterprises demonstrate that the proposed model outperforms traditional baseline models across multiple evaluation metrics, including AUC, Precision, Recall, and F1-score, confirming the effectiveness and practical value of the approach in delinquency risk prediction. This research not only improves the predictive accuracy of risk control models but also offers new insights into modeling complex and heterogeneous credit data.