Deep Attention Model for Sleep Posture Detection Using BCG Signals
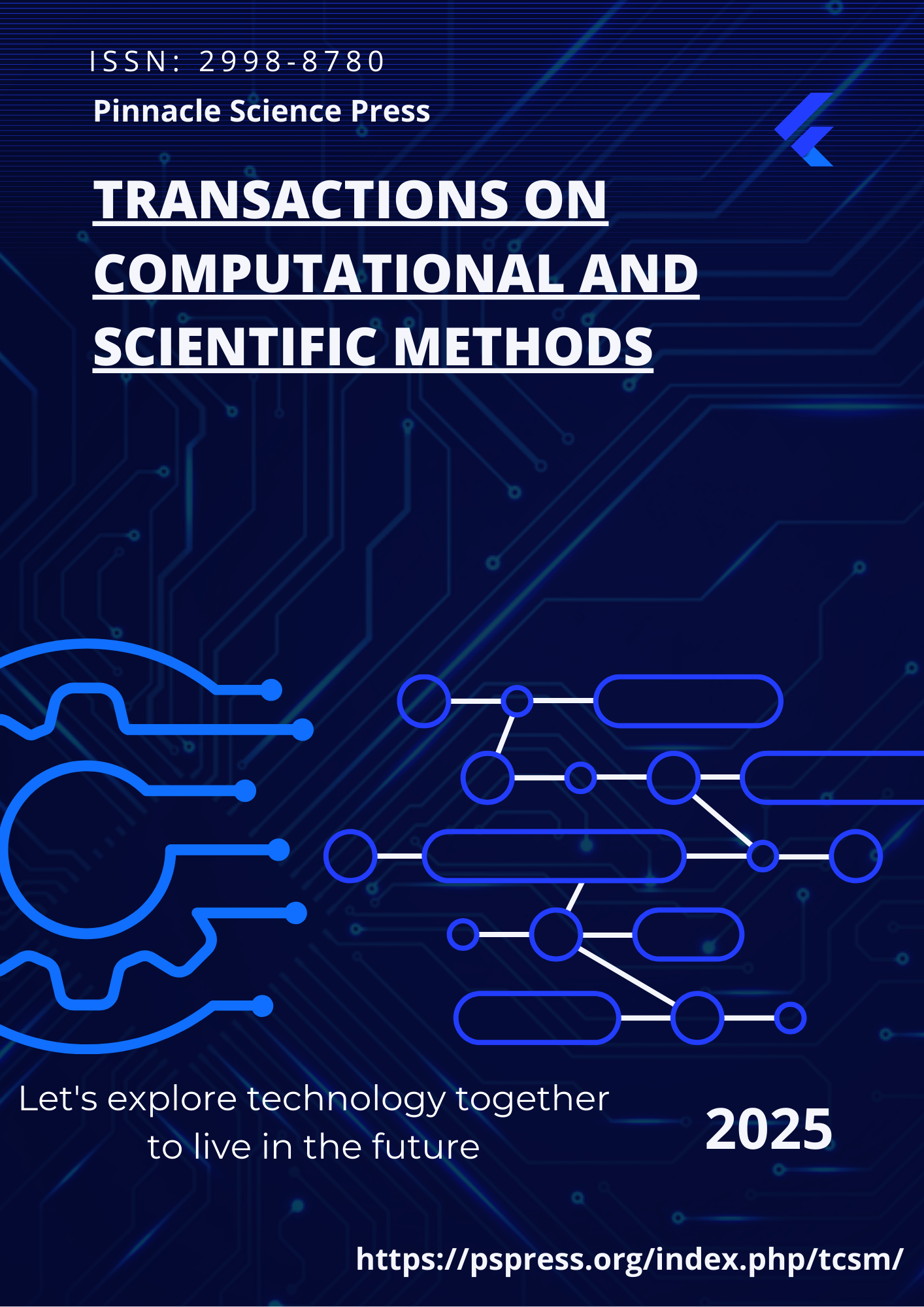
Abstract
This paper proposes a novel deep learning model for non-contact sleeping posture monitoring based on ballistocardiogram (BCG) signals. Traditional methods struggle with BCG signals due to their weak amplitude, non-stationarity, and noise sensitivity. To address these challenges, we introduce a Convolutional and Temporal Attention-based Monitoring model (CTAM) that integrates spatial feature extraction via convolutional neural networks (CNN), temporal modeling through long short-term memory (LSTM) networks, and an attention mechanism for dynamic feature weighting. CTAM is designed as an end-to-end framework capable of real-time classification of four primary sleeping positions (supine, prone, left lateral, and right lateral). Compared to baseline CNN and CNN-LSTM architectures, CTAM demonstrates superior convergence speed and classification accuracy, with improvements of 1.46% and 4.61% on the test set, respectively. These results validate the model’s effectiveness for real-time, non-invasive, and robust sleep posture recognition and suggest strong potential for clinical and consumer-grade sleep monitoring applications.